











|
Tutorial Sessions
Tutorial Co-Chairs:
Professor Jie (Peter) Liu, Carleton University, Ottawa, Canada
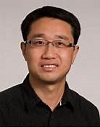
Dr Jamie Coble, University of Tennessee, Knoxville, TN
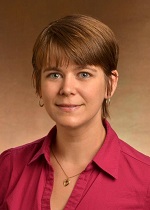
___________________________________________________________________________________________
Tutorial 1: Incipient fault detection and diagnosis using statistical signal processing
Instructors:
Professor Demba Diallo, University of Paris-Sud, France
Professor Claude Delpha, University of Paris-Sud, France
Tutorial Abstract:
The last three decades have shown an increased demand for systems health monitoring due to increasing safety rules and economic reasons. Indeed, in order to avoid unwanted stops it is crucial to accurately detect faults at their early stage. Moreover, the fault severity estimation or assessment and the fault evolution (prognosis) may be requested. In a wide variety of industrial and on-board applications, the fault detection and diagnosis are based on data driven approaches obtained from numerical models or experimental data. Considering such approaches, statistical-based techniques offer many attractive advantages in dealing with large variable sets encountered in complex systems. Among various statistical-based techniques, Principal Component Analysis (PCA)-based ones are the most popular. Their main interests are their ability to reduce the data dimensional-space while keeping the maximum variance information available before the application of different statistical criteria for performing the detection (T2, Q-statistics, and f-divergences). The complexity of this fault detection is more tedious if environmental noise or nuisance parameters mask the fault information and induce detection errors (missed detection and false alarms). The presentation will first define a very small (incipient) fault considering the noise nuisance influence on the signal. Then we will discuss solutions to obtain accurate detection and estimation of such faults considered as hidden information. The performances will be particularly discussed on simulation and experimental vibration signals and impedance measurements from Eddy Current Non-Destructive Evaluation.
Presenters’ Biographies:
Prof. Demba Diallo (IEEE SM’05) received the M.Sc. and Ph.D. degrees both in Electrical and Computer Engineering, from the National Polytechnic Institute of Grenoble, France, in 1990 and 1993 respectively. After graduation, he worked from 1994 to 1999 as a Research Engineer at the Laboratoire d’Electrotechnique de Grenoble, France, on electrical drives and active filters. In 1999, he joined the University of Picardie “Jules Verne” as Associate Professor of Electrical Engineering. He’s currently an Associate Professor of the Univ.Paris Sud and the Group of Electrical Engineering Paris. His area of academic and industrial research includes advanced control techniques and diagnosis of AC drives, renewable energies, design of electric powertrains and autonomous systems. He’s an Editor of the IEEE Transactions on Vehicular Technology and the International Journal on Energy Conversion (IRECON). He is also an Editorial Board Member of ISRN Power Engineering.
Prof. Claude Delpha (M'10) is an Associate Professor in the Université Paris-Sud, France. He graduated in Electrical and Signal Processing Engineering. He obtained his Ph.D. in the Université de Lorraine - Metz in the field of sensors instrumentation and signal processing with a smart sensors system based application. Since 2001, he is with the Laboratoire des Signaux et Systèmes in Gif sur Yvette (south of Paris, France). He works in the field of signal processing for complex systems security and process monitoring (multimedia and smart systems): information detection and estimation His main areas of interests are Multi-dimensional and statistical Signal Processing, Data hiding (watermarking, steganography), Pattern Recognition, Fault Detection and Diagnosis (incipient and intermittent).
___________________________________________________________________________________________
Tutorial 2: Deep Learning for PHM Applications
Instructor: Dr. Weizhong Yan, Principal Scientist, GE Global Research Center, USA
Tutorial Abstract:
Deep learning is a sub-field of machine learning that involves learning good representations of data through multiple levels of abstraction. By hierarchically learning features layer by layer, with higher-level features representing more abstract aspects of the data, deep learning can discover sophisticated underlying structure and features, thus achieving an improved predictive performance. Deep learning, regarded as one of the breakthrough technologies in machine learning in recent years, has attracted tremendous research attention recently in both academia and industrial communities. Companies like Google, Facebook and Apple have heavily invested into this field of research. Recently deep learning has resulted in state-of-the-art performance in many applications, including image and video classification, computer vision, speech recognition, natural language processing, and audio recognition. Deep learning, however, has not been actively applied to the PHM domain. PHM applications deal with data that are primarily sensor measurements. As sensors are prone to different types of faults, such as bias, drift, scaling, and drop out, PHM data are much noisy. Besides data being noisy, PHM applications also involve a wide range of assets that are operated under different physical principals and various operating conditions and are subject to various failure mechanisms/modes. These unique characteristics make the PHM applications significantly differ from other applications, i.e., computer vision and speech recognition, where deep learning has been well studied. How to make deep learning work effective for PHM applications is still an open research question. In this tutorial, I will first provide an overview of deep learning technology. I will then focus on discussing technical strategies and tactics on using deep learning for PHM applications. Finally I will share several my own real-world examples on using deep learning for PHM applications. I hope the tutorial can shed some light on applying deep learning to our PHM domain. Ultimately I hope this tutorial can stimulate more research interests towards deep learning technology within our PHM community.
Presenter’s Biography:
Weizhong Yan, PhD, PE, has been with the General Electric Company since 1998. Currently he is a Principal Scientist in the Machine Learning Lab of GE Global Research Center, Niskayuna, NY. His research interests include neural networks (shallow and deep), big data analytics, feature engineering & feature learning, ensemble learning, and time series forecasting. He specializes in applying advanced datadriven analytic techniques to anomaly detection, diagnostics, and prognostics & health management of industrial assets such as jet engines, gas turbines, and oil & gas equipment. He has authored over 70 publications in referred journals and conference proceedings and has filed over 30 US patents. He is an Editor of International Journal of Artificial Intelligence and an Editorial Board member of International Journal of Prognostics and Health Management. He is a Senior Member of IEEE.
___________________________________________________________________________________________
Tutorial 3: Gas Turbine Performance, Key to Diagnostics
Instructor: Professor Herb Saravanamuttoo, Carleton University, Canada
Tutorial Abstract:
A basic understanding of gas turbine behavior is key to the development of diagnostic systems, this being especially important for users. This presentation will cover the elements of off-design performance, the effect of ambient conditions on engine operation and a brief description of some common types of deterioration.
Presenter’s Biography:
Herb Saravanamuttoo was educated at Allan Glen’s School , Glasgow and the University of Glasgow. After graduation in 1955 he joined Orenda Engines in Canada, where he worked on the development of the Iroquois engine for the Avro Arrow and a family of aero-derivative gas turbines. In 1964 he became a Faculty Member at the University of Bristol, where he worked with Bristol Siddeley ( later Rolls Royce ) and BAC on development of the Olympus 593 and Concorde, and RR on the early development of the RB 211. He obtained his PhD in 1968 and joined Carleton in 1970. He spent 28 years , including 10 years as Chairman of the Dept of Mech and Aerospace Engrg and retired in1998. He was a Founding Director of GasTOPS and has maintained strong contacts with the gas turbine industry on both sides of the Atlantic. He has worked on gas turbine diagnostics for a wide range of aero and industrial gas turbines. He continues to lecture at Carleton and Cranfield and has given many in-house courses at both GT manufacturers and users. He is a Fellow of ASME, IMechE,CASI and the Canadian Academy of Engineering and Past President of CASI. He is co-author of Gas Turbine Theory, now entering its 7 th edition and in continuous print since 1951.
___________________________________________________________________________________________
Tutorial 4: Machine learning-based methods for estimating time to failure with application to APU prognostics
Instructor: Dr. Chunsheng Yang, Senior Research Officer, National Research Council, Canada
Tutorial Abstract:
The need for higher equipment availability and lower maintenance cost is driving the development and integration of prognostic and health management (PHM) systems. Taking advantage of advances in sensor technologies, PHM systems enable a predictive maintenance strategy through continuously monitoring the health of complex systems. The core of PHM technology is prognostics which is able to predict the likelihood of a failure and estimate time to failure (TTF) using the built-in predictive models. However, TTF estimation is still remaining a challenge issue in developing PHM systems. In this talk, the state of the art of TTF estimation will be first reviewed. After introduction of traditional methods of TTF estimation, we will present the novel methods --machine learning-based approaches-- for estimating TTF, including classification, regression, on-demand regression, Particle Filtering (PF)-based method, and so on. In particular, we will elaborate the on-demand regression method developed based on machine learning and data mining techniques. The tutorial will give details on implementation of the algorithms and its application to Auxiliary Power Unit (APU) prognostic.
Presenters’ Biography:
Dr. Chunsheng Yang is a Senior Research Officer at the National Research Council Canada. He is interested in data mining, machine learning, reasoning technologies such as case-based reasoning, rule-based reasoning and hybrid reasoning, intelligent systems, and distributed computing. He received a B.Sc. in Electronic Engineering from Harbin Engineering University, China, a M.Sc. in computer science from Shanghai Jiao Tong University, China, and a Ph.D. from National Hiroshima University, Japan. He worked with Fujitsu Inc., Japan, as a Senior Engineer and engaged on the development of ATM Network Management Systems. He was an Assistant Professor at Shanghai Jiao Tong University from 1986 to 1990 working on Hypercube Distributed Computer Systems. Dr. Yang has been the author for 135 technical papers (book chapters) and reporters published in the referred journals and conference proceedings. He is a Program Co-Chair of the 20th IEEE International Conference on Computer Supported Cooperative Work in Design (CSCWD 2016). He was a Program Co-Chair for the 17th International Conference on Industry and Engineering Applications of Artificial Intelligence and Expert Systems. Dr. Yang is a guest editor for the International Journal of Applied Intelligence. He has served Program Committees for many conferences and institutions, and has been a reviewer for many conferences, journals, and organizations, including Applied Intelligence, NSERC, IEEE Trans., ACM KDD, PAKDD, AAMAS, and IEA/AIE and so on. He is an Adjunct Professor for Nagoya Institute of Technology (Japan), National Ocean University (Taiwan), Chongqing University(China), Beijing Normal University, and Harbin Engineering University. Dr. Yang is a senior IEEE member.
___________________________________________________________________________________________
Tutorial 5: State Estimation Methods for Structural Damage Monitoring, Diagnosis and Prognosis
Instructor: Eric M. Hernandez – Assistant Professor, University of Vermont
Cumulative damage, such as fatigue, can be modeled as part of the state variables describing the dynamic behavior and evolution of a system. Damage monitoring typically involves two aspects: usage monitoring and mechanistic damage modeling. Under known excitations, state-space models can be used to make quantified predictions of the system reliability. In systems operating under uncertain conditions, such as random vibrations, predictions take the form of probabilistic estimates. If measurements of the system response are available, then state estimation methods can be used in near real-time to improve estimates of the hidden state variables, including damage. This tutorial presents dynamic Bayesian state-estimation methods for monitoring cumulative damage in structural systems. The tutorial will describe methods such as linear, extended and unscented Kalman filter, as well more recent methods such as natural model-based state estimators. The tutorial will present a balance of theoretical development, implementation algorithms and applications in structural systems.
Presenter’s bio:
Dr. Eric Hernandez is an assistant professor in the School of Engineering at the University of Vermont. His research is focused on developing mathematical and computational methods to solve inverse problems under uncertainty, with particular interest in stochastic systems. Some of these inverse problems involve model/parameter identification, load monitoring, state estimation and damage detection. His research has found application in structural health monitoring, damage diagnosis and prognosis of different kinds of structures such as bridges, buildings and wind turbines.
His research has been funded by the National Science Foundation and the Vermont Agency of Transportation. In 2015 he received a Faculty Early Career Development award (CAREER) from the National Science Foundation to develop structural health monitoring, diagnosis and prognosis algorithms for minimally-instrumented large scale civil engineering structures susceptible to fatigue damage. Dr. Hernandez holds a BS in Civil Engineering from Universidad Nacional Pedro Henriquez Ureña in Dominican Republic and a PhD from Northeastern University in Boston.
___________________________________________________________________________________
Tutorial 6: Intelligent Condition Based Monitoring of Rotating Machines
Instructor: Nishchal Kumar Verma, IIT Kanpur, India
This tutorial will be based on interesting framework to develop effective sensory data driven CBM models for rotating machines producing acoustic and vibration signals. The content of this tutorial is divided in to three parts. First part will explain Data Acquisitions strategies using various Sensors and performing the primary operations of data pre-processing, Feature extraction, feature selection and classification for fault recognition. Second part will discuss about finding the most salient position on/near the machine for placing Sensor(s) using Sensitive Position Analysis. Optimizing the number of Sensors and finding better locations for placing sensors are major concerns for many PHM applications, especially w.r.t. reliability of diagnostic outcomes and cost efficiency. However, much research is not available to this problem, and the tutorial shall give a brief history as to how this problem was first tackled with statistical analysis and later through computational intelligence techniques. The final and third part will discuss the strategy to make these technologies portable that helps significantly in cutting down labor cost along with increased efficiency. This part will also illustrate how the entire technology as described earlier is implemented for Air compressor monitoring and Drill bit monitoring using PC, tablets and smartphones including the challenges faced in this endeavor.
Presenter’s bio:
Nishchal Kumar Verma received his Ph.D. from IIT Delhi, India, in 2007 in Electrical Engineering. He is currently an Associate Professor with the Department of Electrical Engineering, IIT Kanpur, India and recipient of Devendra Shukla Young Faculty Research Fellowship from IIT Kanpur for 2013-16. He is an IETE Fellow, IEEE Senior Member, and was Chairman of IEEE UP Section Computational Intelligence Society Chapter in 2013. His research interests include diagnosis and prognosis for health management, big data, internet of things, intelligent data mining algorithms, computer vision, and computational intelligence. He authored/co-authored more than 130 research papers in reputed international journals and conferences. Dr. Verma is the Editor of IETE Technical Review, an Associate Editor of the IEEE Computational Intelligence Magazine, Transactions of the Institute of Measurement and Control, UK, and editorial board member for several reputed journals and conferences.
Dr. Verma served as General Chair of IEEE WCI 2015, IEEE WCI 2013 and co-organizer of ACODS 2014. He has also been the Principle Investigator (PI) and Co-PI of more than 14 research projects. Dr. Verma has been actively working in developing manufacturing technologies for The Boeing Company, USA since 2009.
Return to conference program |