










|
Technical program
1) Keynote Speakers:
i. Equipment and Process Health Management within Aerospace Manufacturing Environment
Dr. Al Salour, Boeing Technical Fellow, The Boeing Company, USA
Dr. Al Salour is a Boeing Technical Fellow and the enterprise leader for the Network Enabled Manufacturing technologies. He is responsible for systems approach to develop, integrate, and implement affordable sensor based manufacturing strategies and plans to provide real time data for factory systems and supplier networks. He is building a model for the current and future Boeing factories by streamlining and automating data management to reduce factory direct labor and overhead support, and promote manufacturing as a competitive advantage. Dr. Salour is the research investigator with national and international premiere universities and research labs. He is the industrial advisory board member for the Intelligent Maintenance Systems (IMS) and is a member of Industrial wireless technical working group with the national institute of Standards and Technology. Dr. Salour has 30 invention disclosures, 17 patents and 1 trade secret in manufacturing technologies.
Abstract: Dr. Al Salour who is Boeing’s Technical Fellow in network enabled manufacturing will be speaking about asset reliability and health monitoring within aerospace manufacturing. He will address the needs and methods for data acquisition and performance analysis to determine overall equipment effectiveness and condition based maintenance optimization. Discussions will include failure mode and effects and how future machines can detect anomalies and degradations before system failures occur. Dr. Salour will present roadmaps and models on how advanced factory systems can contribute to productivity and competitive advantages.
ii. Enabling Autonomous Computing – Technology solutions that anticipate and avert failure
Dr. William R. Tonti, IEEE Fellow, Past IEEE Reliability Society President, USA
Dr. Tonti holds a BSEE from Northeastern University, an MSEE and a P.h.D from the University of Vermont, and an MBA from St. Michael’s College. He retired from IBM in 2009 after 30+ years of service, working as the lead semiconductor technologist responsible for IBM’s advanced node development for a large part of his career. Dr. Tonti holds in excess of 290 issued patents, and has been recognized as an IBM Master Inventor. He was honored by having his 250’th patent issue transcribed into the U.S. Congressional Record. Dr. Tonti is recognized as one of the worlds leading inventors, developing over 200 patent families. He is a lead inventor of the modern day embedded electronic fuse.
Dr. Tonti is a Fellow of the IEEE, a past IEEE Reliability Society President, a recipient of the IEEE Reliability Engineer of the Year award, and the IEEE 3’rd Millennium medal. Dr. Tonti joined IEEE in 2009 as the Director of IEEE Future Directions where he works alongside staff and volunteers to incubate new technologies within the IEEE.
Abstract: Current and future computing solutions demand a solution that guarantees zero failures of the architecture through a systems useful life. Traditional technology solutions to guarantee requirements has become difficult as the field use conditions have moved off of a design nominal and approach the maximum allowed. Figure 1 shows a system level instantaneous failure rate as a function of field use power on hours. Region II is the expected intrinsic failure rate during a systems useful life. The issue described in this talk is the compression of Region III to Region I.
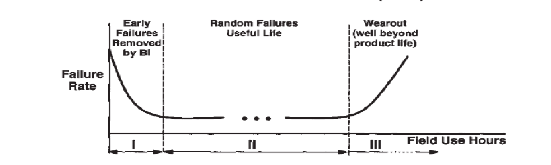
Figure 1: Bathtub curve-Instantaneous system failure rate
Region I: Time "0" extrinsic failures
Region II: Useful life, intrinsic failures
Region III: End of life, Wearout
On chip techniques that anticipate or react to a measured failure through repair solution implementation are the subject of this talk. Figure 2 (USP 7,966,537) describes a topology that anticipates failure and autonomously executes an in system repair using on chip one time programmable e-Fuse (Figure 3). The integration of in die field programmable e-Fuse with on board diagnostics coupled to a repair solution is one method that leads to autonomous computing.
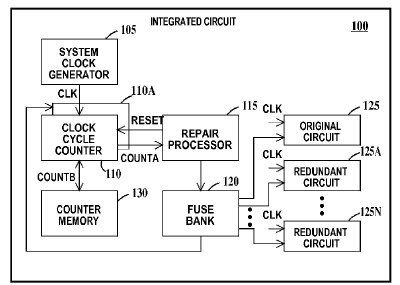
Figure 2: USP7,966,537 Autonomic computing solution for use in an MCIOT implementation
In this example, circuit repair is autonomically enabled based on a use model that tracks active cycles. e-Fuse technology is used to implement the repair by replacing internal elements at their end of life.
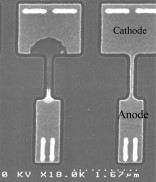
Figure 3: Electronic Fuse (e-fuse) shown in the 90nm Silicon node
Programmed mode (left) and un-programmed mode (right). One time programming is accomplished through controlled high currents that uses the process of electromigration in the e-Fuse. This alters the e-fuse impedance from a low to a high state when programmed.
2) Tutorial Speakers:
Tutorial 1: Fault Diagnostics and Prognostics
Dr. Chiman Kwan, Founder and Chief Technology Officer, Signal Processing Inc., USA
Dr. Chiman Kwan received his BS with honors in Electronics from the Chinese University of Hong Kong in 1988, and MS and Ph.D. degrees in electrical engineering from the University of Texas at Arlington in 1989 and 1993, respectively. He is the founder and Chief Technology Officer of Signal Processing, Inc. and Applied Research LLC, leading research and development effort in real-time control, chemical agent detection, biometrics, speech processing, image fusion, remote sensing, mission planning for UAVs, and fault diagnostics and prognostics.
From April 1991 to February 1994, he worked in the Beam Instrumentation Department of the SSC (Superconducting Super Collider National Laboratory) in Dallas, Texas, where he was heavily involved in the modeling, simulation and design of modern digital controllers and signal processing algorithms for the beam control and synchronization system. He later joined the Automation and Robotics Research Institute in Fort Worth, where he applied intelligent control methods such as neural networks and fuzzy logic to the control of power systems, robots, and motors. Between July 1995 and April 2006, he was the Vice President of Intelligent Automation, Inc. in Rockville, Maryland.
Over the past 20 years, Dr. Kwan has served as Principal Investigator/Program Manager for more than 110 competitively selected projects with total funding more than 35 million dollars from various government agencies and private companies such as Ford, Motorola, Boeing, Honeywell, and Stanford Telecom. He has 13 issued and pending patents, 42 invention disclosures, and 275+ journal and conference papers. He is listed in the New Millennium edition of Who’s Who in Science and Engineering. He served as the Treasurer of Control Systems Society Chapter in IEEE NoVa section in 2016 and is currently the Chair of that chapter.
Abstract: This tutorial will provide an introduction to fault diagnostics and prognostics algorithms and their applications. There will be two parts:
Diagnostic:
- Model based methods (state space)
- Data driven methods (PCA, nonlinear PCA, neural networks, support vector machine)
- Applications: sensor validation, actuator fault isolation, fault tolerant control, fault location in power network, helicopter gearbox monitoring, etc.
Prognostic:
- Model based methods (damage mechanics, etc.)
- Data driven methods (HMM, PCA)
- Applications: bearing and gearbox remaining life prediction, intermetten failure prediction in digital electronics, etc.
Tutorial 2: Dimensionality Reduction Theories for PHM
Dr. Yixiang Huang, Assistant Professor, Shanghai Jiaotong University, China
Dr. Yixiang Huang iis an Assistant Professor in the Department of Mechanical Engineering at Shanghai JiaoTong University, China. Previously, he worked from 2010 to 2012 as a researcher and postdoctoral fellow at the NSF Industry/University Cooperative Research Center for Intelligent Maintenance Systems at the University of Cincinnati, USA. His current research interests include intelligent maintenance, prognostics and health management, dimensionality reduction techniques, big data analysis and sparse coding for various applications. He has applied advanced data driven techniques in fault diagnosis, anomaly detection, prognostics and health management not only to the industrial assets such as construction and mining equipment, machine centers, assembling production line, mixshield machine, wind turbines, batteries, robots, but also to the non-industrial applications such as electroencephalography (EEG) and electrocardiogram (ECG) signals for human health assessment. He has authored over 40 publications in referred journals and conference proceedings. He has served as the paper review chairs, session chairs in several international conferences such as COMADEM, International Conference on Sensing, Diagnostics, Prognostics, and Control (SDPC), etc.
Abstract: This tutorial will start with a brief review of the dimensionality reduction techniques, with some of the key concepts associated with data analysis and visualization, for applications of both linear and non-linear data sets, also for time sensitive networks and storage sensitive networks (e.g., IoT, edge computing, big data). This will be followed by several case studies and a quick hands-on part with exercises where participants will be able to practice some popular dimension reduction techniques in sample scenarios. Next, the tutorial will also introduce the development trend of the dimension reduction techniques and some of the open questions in real-world applications. This tutorial will benefit PHM researchers who work with large number of variables or features, such as analysts, scientists, data engineers, data scientists, algorithm developers, and students or teachers.
Tutorial 3: Data Driven Models for Condition Based Monitoring of Machines
Dr. Nishchal K. Verma, Associate Professor, Indian Institue of Technology, Kanpur, India
Dr. Nishchal K. Verma (SM’13) is an Associate Professor in Dept. of Electrical Engineering, Indian Institute of Technology Kanpur, India. He received his PhD in Electrical Engineering from Indian Institute of Technology Delhi, India. He worked as Post-Doctoral Research Fellow in Center for Integrative and Translational Genomics, University of Tennessee, Memphis, TN 38163 USA and Post-Doctoral Research Associate in Department of Computer Science, Louisiana Tech University, Ruston LA 71270 USA. He was awarded Devendra Shukla Young Faculty Research Fellowship by Indian Institute of Technology Kanpur, India for year 2013-16 and recently, he is awarded Achiever award by Institution of Engineers at Jodhpur, India on Engineers day (Sept. 15th, 2017).
His research interests include Cognitive Science, Big Data, Bioinformatics, Intelligent Data Mining Algorithms and Applications, Computer Vision and Image Processing, Brain Computer/Machine Interface, Machine Intelligence, Intelligent Informatics, Smart Grid, Intelligent Fault Diagnosis Systems, Prognosis and Health Management, Soft-Computing in Modelling and Control, Internet of Things/ Cyber Physical Systems, Intelligent Agents and their Applications, Fuzzy Logic and Controllers, Deep Learning of Neural and Fuzzy Networks, Machine Learning Algorithms, Computational Intelligence. He authored and co-authored more than 190 research papers.
Dr. Verma is an IETE Fellow. He is currently serving as an Editor of the IETE Technical Review Journal, an Associate Editor of the IEEE Computational Intelligence Magazine, an Associate Editor of the Transactions of the Institute of Measurement and Control, U.K., Guest Editor of special issue on “Computational Intelligence” of International Journal of Swarm Intelligence, Guest Editor of special issue on “Computational Intelligence: Theories, Applications and Future Directions” of International Journal of Artificial Intelligence and Soft Computing and an Editorial Board Member for several journals and conferences. Abstract: Intelligent health monitoring of machines using data driven approaches are designed to develop a real time health monitoring system which can continuously monitor the status of various components of machines and notify as soon as anomaly is detected. Data driven approaches are normally based on machine learning techniques and are largely dependent on signal pre-processing, feature extraction, feature selection and classification modules. Usually, large number of features are obtained in feature representation. In which some of them are irrelevant and does not contain useful information about the machine condition. Therefore, these features should be minimized to increase the classification accuracy of the classifier.
This tutorial starts with introduction to data acquisition, sensitive position analysis, data pre-processing, feature extraction, feature selection, and classification for condition-based health monitoring of machines.
Tutorial 4: Analytics at Boeing and a Prognostic Example
Dr. Zhennong Wang, Associated Technical Fellow, Boeing Global Services, The Boeing Company, USA
Dr. Zhennong (Michael) Wang is an Associate Technical Fellow at the Boeing Company, and currently Dr. Wang is with Digital Aviation & Analytics of Boeing Global Services, a newly created division in Boeing focusing on providing aftermarket services. Dr. Wang earned his Ph.D. degree in applied mathematics from the University of Kansas. Currently his interests focus on big data, machine learning and AI, and using airplane maintenance data to conduct airplane parts reliability analysis and modeling.
Abstract: In Boeing’s 100 year history, Boeing has collected a lot of airplane data, from airplane design, airplane manufacturing, airplane operation, logistic support to airplane reliability. In order to answer business challenges and better utilize the data to support airlines, Boeing created the Analytics group. In this tutorial, an introduction to Boeing AnalytX and an example of using airplane part operation data to conduct prognostic analysis will be presented.
Boeing has a long history using data analytics to improve product design and better serve airline customers. A history review of data analytics, the capabilities of AnalytX, and how Boeing uses analytics to bring values to airlines will be presented.
When people conduct reliability analysis, one of challenges is to get data related to a product, particularly to get operation data while the product is operating at customer sites. In order to better serve airline customers, Boeing, airline customers and suppliers have come up to an industry program—ISDP, and agreed to collect airplane part failures and removals data to improve airplane dispatch reliability and airplane design. In this presentation, a method on how to get airplane parts’ time-on-wing data, using math models to model the life data, and using the results to make prognostic health and survivability of airplane parts will be discussed.
Tutorial 5: New Developments on Diagnostics and Health Management Applied to Unmanned Systems
Dr. Youmin Zhang, Professor, Department of Mechanical, Industrial and Aerospace Engineering, Concordia University, Canada
Bio: Youmin Zhang received the B.S., M.S., and Ph.D. degrees from Northwestern Polytechnical University, Xi'an, China, in 1983, 1986, and 1995, respectively. He is currently a Professor with the Department of Mechanical, Industrial and Aerospace Engineering and the Concordia Institute of Aerospace Design and Innovation, Concordia University, Montreal, Quebec, Canada.
His current research interests include condition monitoring, health management, Fault Detection and Diagnosis (FDD), and Fault-Tolerant Control Systems (FTCS), cooperative Guidance, Navigation, and Control (GNC) of single and multiple unmanned aerial/space/ground/surface vehicles and their applications to forest fires, pipelines, power lines, environment, natural resources and natural disasters monitoring, detection, and protection by combining with remote sensing techniques; dynamic systems modeling, estimation, identification, advanced control techniques and signal processing techniques for diagnosis, prognosis, and health management of safety-critical systems, renewable energy systems and smart grids, and intelligent manufacturing processes. He has authored 4 books, over 460 journal and conference papers, and book chapters. He is a Fellow of Canadian Society of Mechanical Engineering (CSME), a Senior Member of the American Institute of Aeronautics and Astronautics (AIAA) and the Institute of Electrical and Electronics Engineers (IEEE), and a member of the Technical Committee (TC) for several scientific societies, including the International Federation of Automatic Control (IFAC) Technical Committee on Fault Detection, Supervision and Safety for Technical Processes, the AIAA Infotech@Aerospace Program Committee on Unmanned Systems, the IEEE Robotics and Automation Society TC on Aerial Robotics and Unmanned Aerial Vehicles, the ASME/IEEE TC on Mechatronics and Embedded Systems and Applications, and the International Conference on Unmanned Aircraft Systems (ICUAS) Association Executive Committee. He has been invited to deliver plenary and tutorial talks at international conferences/workshops and research seminars worldwide for over 90 times. He is a founding Editor-in-Chief of the Journal of Instrumentation, Automation and Systems, an Editor-at-Large of the Journal of Intelligent & Robotic Systems, and an Editorial Board Member/Associate Editor of several other international journals (including three newly launched journals on Unmanned Systems). He has served as General Chair, Program Chair, Program Vice Chair, and IPC Member of many international conferences, including the General Chair of the 10th International Conference on Intelligent Unmanned Systems (ICIUS) in 2014, Montreal, Canada, Program Chair of the International Conference on Unmanned Aircraft Systems (ICUAS) in 2014, Orlando, FL, USA, one of General Chairs of the ICUAS in 2015, Denver, USA, a Co-General Chair of the ICIUS 2016 to be held at Xian, China, Program Chair of the ICUAS 2017 held at Miami, USA, a General Co-Chair of the 2nd Int. Symp. on Autonomous Systems (ISAS’18), Chongqing, May 19-21, 2018, and General Chair of the ICUAS 2018 to held at Dallas, USA in June 12-15, 2018. More detailed information can be found at http://users.encs.concordia.ca/~ymzhang/.
Abstract: Unmanned Systems (USs) including Unmanned Aerial Vehicles/Systems (UAVs or UASs), Unmanned Ground Vehicles (UGVs) and Autonomous/Driverless Vehicles, as well as Unmanned Surface/Underwater Vehicles (USVs/UUVs) are gaining more and more attention during the last a few years due to their important contributions and cost-effective applications in several tasks such as surveillance, sensing, search and rescue, agriculture, forest and environment, pipelines and powerlines, military and security applications. On the other hand, Diagnostics and Health Management (DHM) of USs have also been attracted more and more attention due to the requests of safety and reliability of using these USs for the above-mentioned various applications. In this talk, the DHM issues will be represented in detail as functions of Fault Detection and Diagnosis (FDD) and Fault-Tolerant Control (FTC). Benefited from the recent and significant advances and developments of USs, new developments on FDD, FTC, and even newly developed Fault-Tolerant Cooperative Control (FTCC) techniques have been emerged and developed quickly in recent years. In this talk, brief review on the recent developments of autonomous unmanned systems and challenges on diagnostics (FDD) and health management (FTC, FTCC) of unmanned systems will be given, then the new developments and current research works on the FDD, FTC and FTCC techniques with applications to autonomous quadrotor UAVs, wheeled mobile robots/ground vehicles and unmanned surface vehicles testbeds developed in collaboration with industry at Concordia University, as well as applications to wind turbines/farm and CNC machine tools, will be introduced.
3) Panel Talks:
Deep Learning in Prognostic and Health Management:
Prognostics and health management (PHM) is a multi-disciplinary research area that provides efficient and robust solutions for managing the health of machines mainly in an industrial environment. For past few years, PHM has been catering the needs of engineering community in industries and academics for developing methodologies to achieve the objectives of PHM such as reliability, maintainability, safety, and affordability of machines in an industrial environment. In the recent years, a large number of machine learning based approaches have been suggested to achieve the objectives of the PHM. However, with the help of deep learning strategies, which is an advanced learning technique, the performance of machine learning/computational intelligence based models can be greatly improved for better PHM of the machines under uncertain and noisy environment. Some of the deep learning based models, beings applied in PHM are deep neural networks (DNN), deep belief network (DBN), deep Boltzmann machine (DBM) and deep fuzzy network (DFN) etc.
Dr. Nishchal K. Verma (SM’13) is an Associate Professor in Dept. of Electrical Engineering, Indian Institute of Technology Kanpur, India. He received his PhD in Electrical Engineering from Indian Institute of Technology Delhi, India. He worked as Post-Doctoral Research Fellow in Center for Integrative and Translational Genomics, University of Tennessee, Memphis, TN 38163 USA and Post-Doctoral Research Associate in Department of Computer Science, Louisiana Tech University, Ruston LA 71270 USA. He was awarded Devendra Shukla Young Faculty Research Fellowship by Indian Institute of Technology Kanpur, India for year 2013-16 and recently, he is awarded Achiever award by Institution of Engineers at Jodhpur, India on Engineers day (Sept. 15th, 2017).
His research interests include Cognitive Science, Big Data, Bioinformatics, Intelligent Data Mining Algorithms and Applications, Computer Vision and Image Processing, Brain Computer/Machine Interface, Machine Intelligence, Intelligent Informatics, Smart Grid, Intelligent Fault Diagnosis Systems, Prognosis and Health Management, Soft-Computing in Modelling and Control, Internet of Things/ Cyber Physical Systems, Intelligent Agents and their Applications, Fuzzy Logic and Controllers, Deep Learning of Neural and Fuzzy Networks, Machine Learning Algorithms, Computational Intelligence. He authored and co-authored more than 190 research papers.
Dr. Verma is an IETE Fellow. He is currently serving as an Editor of the IETE Technical Review Journal, an Associate Editor of the IEEE Computational Intelligence Magazine, an Associate Editor of the Transactions of the Institute of Measurement and Control, U.K., Guest Editor of special issue on “Computational Intelligence” of International Journal of Swarm Intelligence, Guest Editor of special issue on “Computational Intelligence: Theories, Applications and Future Directions” of International Journal of Artificial Intelligence and Soft Computing and an Editorial Board Member for several journals and conferences.
Big Data in PHM:
Panel Moderator: Dr. Janet (Jing) Lin, Lulea University of Technology, Sweden
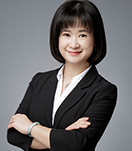
Panelists: Dr. Diego Galar, Dr. Lishuai Li, Dr. Zongchang Liu
Dr. Diego Galar
Short Bio: Dr. Diego Galar is Professor of Condition Monitoring in the Division of Operation and Maintenance Engineering at LTU, Luleå University of Technology where he is coordinating several H2020 projects related to different aspects of cyber physical systems, Industry 4.0, IoT or industrial Big Data. He was also involved in the SKF UTC centre located in Lulea focused on SMART bearings. He is also actively involved in national projects with the Swedish industry and also funded by Swedish national agencies like Vinnova. He is also principal researcher in Tecnalia (Spain), heading the Maintenance and Reliability research group with the Division of Industry and Transport. He has authored more than four hundred journal and conference papers, books and technical reports in the field of maintenance, working also as member of editorial boards, scientific committees and chairing international journals and conferences and actively participating in national and international committees for standardization and R&D in the topics of reliability and maintenance. In the international arena, he has been visiting Professor in the Polytechnic of Braganza (Portugal), University of Valencia and NIU (USA) and the Universidad Pontificia Católica de Chile. Currently, he is visiting professor in University of Sunderland (UK), University of Maryland (USA), and Chongqing University in China.
Title: Virtual commissioning for PHM services: Hybrid digital twins in railway
Dr. Lishuai Li
Short Bio: Dr. Lishuai Li is an Assistant Professor in the Department of Systems Engineering and Engineering Management at City University of Hong Kong (CityU). She is interested in innovative methods for the design, management, operation of transportation systems, drawing expertise in Big Data and Information Technology. She received a Ph.D. and a M.Sc. in Air Transportation Systems from the Department of Aeronautics and Astronautics at Massachusetts Institute of Technology. She received a B.Eng. in Aircraft Design and Engineering from Fudan University. Before joining CityU, she was a consultant at McKinsey & Company in San Francisco.
Title: Monitoring the Health Status of High-Speed Trains via Multi-location Vibration Data: Wheel Wear and Suspension System Degradation
Dr. Zongchang Liu
Short Bio: Dr. Zongchang Liu is the CTO at CyberInsight Co. Ltd. and a PhD Researcher at University of Cincinnati Center for Intelligent Maintenance Systems (IMS). He received his bachelor degree from University of Michigan in Mechanical Engineering, and Shanghai Jiaotong University in Electrical Engineering. His research experience includes developing PHM systems for rotatory machines, high-speed train systems, wind turbine, cargo ships, and battery systems. In the area of railway equipment PHM, his research experience includes induction motor, axial bearing, wheel profile, railway track, and gearbox. He is also a leading developer for WindInsightTM (wind farm smart O&M platform) and CSSC SOMSTM (Smart-ship Operation and Maintenance System) platform. He also has 7 patents in EV battery smart mobility and health management applications, and is the co-author/editor for 4 books in Industrial Big Data and Cyber-Physical Systems.
Title: Industrial AI augmented smart operation and maintenance for industrial assets
4) Special Sessions Information:
- PHM for Transportation. Focusing on PHM applications of sustainable transportation, with a goal of integrating Operational Technology, Information Technology, and Engineering Technology for intelligent transportation systems through effective PHM implementation. Organizer: Dr. Janet Lin janet.lin@ltu.se, Luleå University of Technology (LTU), Luleå, Sweden
- Advanced Signal Processing for PHM. Focusing on the advancement of signal processing methods and applications in PHM. Organizers: Drs. Zhiliang Liu zhiliang_liu@uestc.edu.cn, University of Electronic and Science Technology of China; and Yongbo Li, yongbo@nwpu.edu.cn, Northwestern Polytechnical University, China
- Some Recent PHM Advances and Applications in Aerospace Engineering. Focusing on the recent development of methods and applications of PHM in the aerospace industry. Organizers: Dr. Shuguang Song shuguang.song@boeing.com, The Boeing Company, USA; Prof. Min Xie, minxie@cityu.edu.hk, City University of Hong Kong, Hong Kong.
- AI for PHM:Focusing on the latest developments and implementations of artificial intelligence and data mining methods for PHM. Organizer: Dr. Yan-Fu Li, liyanfu@tsinghua.edu.cn, Tsinghua University, Beijing, China.
- PHM for ultra-high reliability electronics: Focusing on the innovative PHM methods which could minimize the built-in tester’s effects to reliability of ultra-high reliability electronics. Organizer:Dr. Gang Dai, daigang@mtrc.ac.cn, Microsystem and Terahertz Research Center, China Academy of Engineering Physics, Chengdu, China.
- PHM for mission critical complex system: Focusing on new principles, theories, and applications as well as the design of systemic PHM for mission critical complex system. Organizer: Dr. Xiaojian Yi, yixiaojianbit@sina.cn, and Hui-na Mu, and Hai-ping Dong, China North Vehicle Research Institute, Beijing Institute of Technology, Beijing, China
If you would like to organize a special paper session or panel, please send a summary about the proposed session/ panel as well as potential speakers to the Program Chair Dr. Steven Li, zhaojun.li@wne.edu. Thank you for your contributions and look forward to seeing you in Seattle in June 2018. |